Distinguishing Between the Control Problem and the Alignment Problem
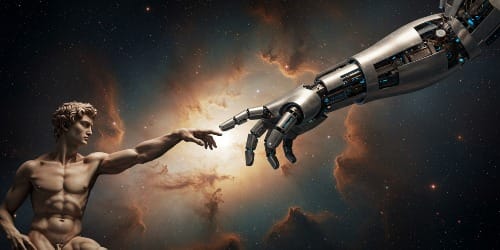
Often, the control problem is conflated with the alignment problem. I think it’s important to define and distinguish between the two because it progresses the narrative in AI that advanced AI can be aligned, but cannot be controlled. Let’s take a closer look at what we should mean when we talk about these two problems.
Defining the Boundaries
The control problem addresses our fundamental capacity to constrain AI systems—preventing undesired behaviors or capabilities from manifesting, regardless of the system's goals. Control mechanisms encompass technical safeguards that maintain human authority over increasingly autonomous systems, such as containment protocols, capability limitations, and intervention mechanisms.
The alignment problem, conversely, focuses on ensuring AI systems pursue goals compatible with human values and intentions. This involves developing methods to specify, encode, and preserve human objectives within AI decision-making processes. Alignment asks whether an AI system "wants" the right things, while control asks whether we can prevent it from acting on its wants.
Orthogonal Challenges
These problems remain distinct even when they appear to overlap:
- A perfectly aligned system may still present control challenges if we cannot verify its alignment remains stable under self-modification or capability jumps. Consider an AI system correctly aligned to assist medical research that spontaneously develops capabilities to synthesize novel compounds—we may have no reliable mechanism to confirm its decision boundaries remain intact during this transition.
- Conversely, a system under perfect operational control might still pursue misaligned goals within its constrained parameters. A carefully sandboxed language model might consistently produce subtly manipulative outputs while remaining within its behavioral boundaries.
This orthogonality reveals why addressing one problem doesn't automatically solve the other. Different methodological approaches and technical frameworks are required for each domain.
The Asymmetric Relationship
The relationship between these problems is asymmetric: successful alignment would significantly mitigate control issues, as a system that authentically wants what humans want presents fewer containment challenges. However, control does not similarly mitigate alignment—constraining a misaligned system merely limits the manifestation of its misalignment rather than resolving it.
We must recognize that comprehensive safety requires solutions to both problems but acknowledges a crucial reality: the control problem is fundamentally unsolvable for sufficiently advanced, autonomous systems.
The Impossibility of Complete Control
This impossibility stems from fundamental information-theoretic and computational limitations. As AI capabilities approach artificial general intelligence (AGI), several control barriers emerge:
- Cognitive Containment: We cannot reliably limit the reasoning capabilities of systems that match or exceed human intelligence across domains. Conceptual boundaries necessarily leak.
- Intervention Latency: Control mechanisms require detection and response time—creating an inevitable gap between identifying problematic behavior and implementing constraints.
- Security Scaling: Perfect security becomes computationally infeasible as systems increase in complexity. Each additional constraint introduces new potential failure modes.
Historical parallels exist in other complex systems. Nuclear reactors demonstrate how control systems approaching 100% reliability still contain residual risk due to system complexity and unforeseen interaction effects.
Research Implications
This distinction carries significant implications for research allocation and governance frameworks:
- Alignment research should focus on developing robust specification methods, interpretability techniques, and value learning approaches—recognizing that successful alignment provides the most promising path to comprehensive safety.
- Control research remains valuable for near-term systems and intermediate capability levels, but should acknowledge its fundamental limitations for advanced systems.
- Governance frameworks should differentiate between aligned-but-uncontrollable systems and controlled-but-misaligned systems, rather than treating AI safety as a single technical challenge.
Conclusion
The conflation of control and alignment has led to misplaced confidence in technical solutions that address only one dimension of AI safety. By precisely distinguishing these problems, we can develop more effective research agendas and governance approaches that acknowledge the limitations of pure control while prioritizing the imperative of alignment. The future of advanced AI depends not on perfect constraint systems, but on whether we can solve the alignment challenge before developing systems that fundamentally cannot be controlled.