Generative AI Poses No Existential Threat
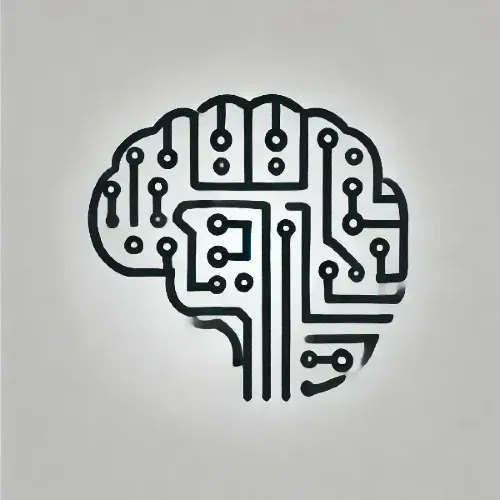
Recent research has transformed our understanding of how artificial intelligence actually learns and develops. Through extensive testing at TU Darmstadt and the University of Bath, researchers have uncovered something fascinating about AI capabilities that challenges our previous assumptions about machine intelligence.
At the heart of this discovery lies a fundamental question: Do advanced AI systems truly develop new abilities spontaneously, or are they simply becoming more sophisticated at learning from examples? The answer, it turns out, reshapes our entire perspective on machine learning.
The researchers conducted over 1,000 experiments, meticulously examining how AI systems learn and develop capabilities. They focused particularly on something called in-context learning—the ability to learn from examples provided within instructions. What they found was illuminating: most abilities I thought emerged spontaneously actually came from this process of learning from examples.
When the researchers removed these contextual examples, something remarkable happened. Most of the supposedly "emergent" abilities disappeared completely. Only two capabilities proved genuinely novel: understanding grammar in nonsense words and recalling specific cultural information about Hinduism. Every other advanced capability stemmed from the AI's sophisticated pattern recognition and ability to learn from examples.
This discovery carries profound implications for AI development and safety. It suggests that AI systems are more predictable than I previously thought. Rather than developing mysterious new capabilities, they excel at pattern recognition and applying learned principles—much like a sophisticated learning system rather than a spontaneously evolving intelligence.
The future of AI development now appears clearer and more directed. Instead of hoping for spontaneous emergence of new abilities, we can focus on enhancing fundamental learning mechanisms. This means more controlled development, better safety protocols, and clearer paths to advancing AI capabilities.
These findings don't diminish the impressiveness of AI systems. Rather, they provide a deeper understanding of how these systems actually work. By recognizing that AI capabilities stem from sophisticated pattern recognition rather than mysterious emergence, we can develop more effective approaches to advancing artificial intelligence while maintaining better control over its development.
The research opens new possibilities for AI development: more focused training methods, better understanding of system limitations, and improved safety measures. Most importantly, it suggests that we can advance AI technology in ways that remain both powerful and predictable.
This shift in understanding marks a crucial moment in AI research. It moves us from a place of uncertainty about spontaneous emergence to a position of greater comprehension and control. The path forward involves enhancing fundamental learning mechanisms rather than waiting for mysterious new capabilities to appear.
- Sheng Lu, Irina Bigoulaeva, Rachneet Sachdeva, Harish Tayyar Madabushi, and Iryna Gurevych. 2024. Are Emergent Abilities in Large Language Models just In-Context Learning?. In Proceedings of the 62nd Annual Meeting of the Association for Computational Linguistics (Volume 1: Long Papers), pages 5098–5139, Bangkok, Thailand. Association for Computational Linguistics.